HADOOP
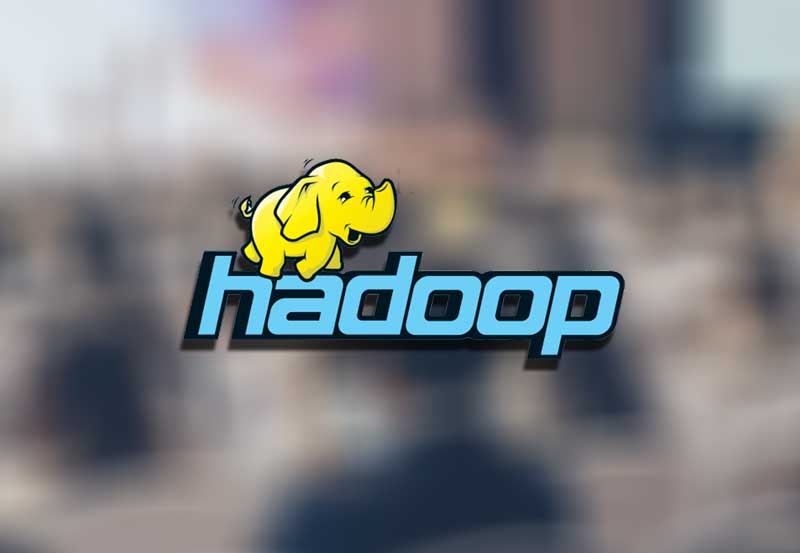
Trainer's Profile
Professionally qualified with more than 12 Years of experience and an extensive BigData experience leading diversified teams spread across different geographical locations.
Training Approach:
The training approach in considereing the following.
- 1. Deep explination of Concept to lay strong foundation.
- 2. Application of concept to a close real time environment with examples of real time use cases.
- 3. Explination of all the possible certification and near possible interview questions.
HADOOP Course Contents
Big Data & Hadoop:
- 1. What is Big Data
- 2. Sources of Big Data
- 3. IBM Definition for Big Data
- 4. Definition of Hadoop
- 5. History of Hadoop
- 6. Features of Hadoop
- 7. Hadoop Eco-System
- 8. Other Hadoop related products of Apache.
Hadoop Distributed File System:
- 1. Distributed File System
- 2. Definition of HDFS
- 3. Where not to use HDFS
- 4. HDFS Concepts
- 5. Hadoop Architecture
- 6. NameNode, DataNode & SNN
- 7. HDFS Federation
- 8. HDFS High Availability
- 9. Hadoop IO Operations(Read & Write)
- 10. HDFS Rack Awareness
- 11. Hadoop Modes
- 12. Hadoop Configuration
- 13. Linux & Hadoop Commands
Java:
- 1. OOP and Java
- 2. Object Oriented Concepts
- 3. Language Fundamentals
- 4. Inheritance
- 5. Polymorphism
- 6. Interface
- 7. Collections
- 8. Exceptions
- 9. Multi Threadings
MapReduce:
- 1. What is MapReduce & Key Value Concepts
- 2. Traditional Solution
- 3. MapReduce Solution
- 4. Input & Output of M/R
- 5. MapReduce Phases
- 6. Anatomy of MapReduce
- 7. WordCount FlowChart
- 8. Advantages of MapReduce
- 9. Input Split in M/R
- 10. Box Classes in Hadoop
- 11. Execution of WordCount Program
- 12. Combiner
- 13. Partitioner
- 14. MapReduce Joins
- 15. Distributed Cache
- 16. Counters
- 17. MapReduce Formats(Input & Output)
YARN:
- 1. Challenges in Hadoop 1.x
- 2. Hadoop 2.x Features
- 3. Apache YARN
- 4. Hadoop 2.x Eco-system
- 5. Hadoop 2.x High Availability
- 6. Anatomy of YARN Application Run
- 7. Run a MapReduce application on YARN
Hive:
- 1. Applications of Hive
- 2. Advantages & Disadvantages of Hive
- 3. Hive Installation & Invoking
- 4. Hive Metastore
- 5. Hive Architecture
- 6. Hive Concepts
- 7. Hive Data Types
- 8. Demonstration of DataBase Commands
- 9. Hive Tables
- 10. Demonstration of Create, rename, alter & Drop
- 11. Partitions in Hive
- 12. Bucketing in Hive
- 13. Hive Joins
- 14. Complex Data Types
- 15. Demonstration of External Table
- 16. SubQueries
- 17. Views
- 18. User Defined Functions (UDFs)
PIG:
- 1. Need for PIG
- 2. PIG versus MapReduce
- 3. Where to use PIG
- 4. Where NOT to use PIG
- 5. What is PIG
- 6. Applications of PIG
- 7. PIG Installation
- 8. Execution Types
- 9. Running PIG programs
- 10. PIG data types
- 11. RDBMS Vs Pig
- 12. Comments in Pig
- 13. Case Sensitivity in Pig
- 14. Logical and Physical Plan
- 15. Pig Operators
- 16. Pig Built in Functions
- 17. Diagnostic Operators in PIG
- 18. Special Joins in PIG:
- 19. Parameter Substitution in PIG
- 20. PIG UDFs
- 21. Pig Best Practices
HBASE:
- 1. What is HBASE & NOSql
- 2. History
- 3. Installation
- 4. Invoke HBase
- 5. HBASE Vs RDBMS
- 6. Uses of HBase
- 7. Where Not to Use HBase
- 8. HBase Write Path
- 9. HBase Read Path
- 10. HBase Terminology
- 11. Row Vs Column Oriented DB
- 12. HBase Architecture
- 13. Data loading Techniques in HBase
- 14. HBase Shell Commands
- 15. Demonstration of HBase shell Commands
Sqoop:
- 1. Introduction and Installation
- 2. Sqoop Tools
- 3. Sqoop Connectors
- 4. Creating a DB and table in MySql
- 5. Loading the MySql DB
- 6. Sqoop Import Process
- 7. Import Hive Data
- 8. Sqoop Export Process
Flume:
- 1. Introduction
- 2. Applications of Flume
- 3. Advantages of Flume
- 4. Features of Flume
- 5. Data Transfer in Hadoop
- 6. Apache Flume - Architecture
- 7. Components of Flume
- 8. Installation of Flume
- 9. Fetching Data using Flume.
Spark:
- 1. MapRedue Vs Spark
- 2. Apache Spark - By Definition
- 3. Features of Spark
- 4. Spark Deployment
- 5. Spark Core & Components
- 6. Spark Context & Invoking
- 7. Prerequisites for Spark
- 8. Resilient Distributed Datasets (RDDs)
- 9. RDD Operations
- 10. RDD Persistence
- 11. Lazy Evaluation & Lineage Graph
- 12. Spark SQL
- 13. Spark SQL Capabilities
- 14. SchemaRDD, DataFrame & Datasets
- 15. Linking with SparkSQL
- 16. Initializing Spark SQL
- 17. SQL Method
- 18. Creating a DataFrame
- 19. Transformations, Actions, Laziness
- 20. Spark Streaming
- 21. Input Sources and Batches of Input
- 22. Discretized Streams
- 23. Initializing StreamingContext
- 24. Transformation on DStreams
- 25. Output Operations on DStreams
- 26. Machine Learning
- 27. Spark MLlib
- 28. Sparks MLlib Packages
- 29. MLlib Data Types
- 30. Spark MLlib Algorithms
- 31. Spark GraphX
- 32. Graph - Getting Started
- 33. Graph Operators
- 34. Graph Builder
- 35. Graph Algorithms
Scala:
- 1. Why Scala
- 2. Functional programming & First Scala Program
- 3. Data Types
- 4. Variable
- 5. Classes
- 6. Objects
- 7. Access Modifies
- 8. Operators, Conditional Statement
- 9. Functions
- 10. Closures