Data Science with Python
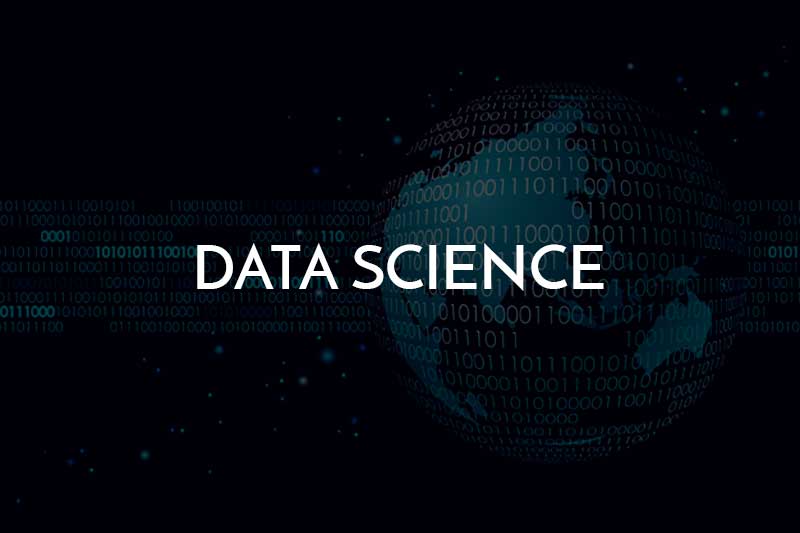
DATA SCIENCE WITH PYTHON
Introduction to Data Science
- 1. What is Data Science?
- 2. Importance of data science
- 3. Demand for Data Science Professional
- 4. Data Science Life cycle
- 5. Tools and Technologies used in data science.
- 6. Roles and Responsibilities of a Data Scientist
COURSE 1: STATISTICS FOR DATASCIENCE
- 1. Module A: Introduction to Statistics
- a. Statistics in Business
- b. Types of Data
- c. Data Measurement Scales
- d. Fundamentals of Probability
- 2. Module B: Descriptive Statistics
- a. Measures of central tendency (Mean, Median and Mode)
- b. Measure of dispersion/spread (Variance and Standard Deviation)
- c. Kurtosis and Skewness
- d. Types of Probability Distributions
- 3. Module C: Inferential Statistics
- a. What is inferential statistics
- b. Different types of Sampling techniques
- c. Central Limit Theorem
- d. Point estimate and Interval estimate
- e. Creating confidence interval for population parameter
- f. Characteristics of Z-distribution and T-Distribution
- 4. Module D: Hypothesis Testing
- a. Basics of Hypothesis Testing
- b. Type of test and Rejection Region
- c. Type of errors-Type 1 Error and Type 2 Errors
- d. Parametric vs Non-Parametric Testing
- e. ANOVA and Chi-Square testes
- 5. Module E: Correlation & Regression
- a. Introduction to Regression
- b. Type of Regression
- c. Correlation
- d. Weak and Strong Correlation
COURSE 2: PYTHON FOR DATA SCIENCE
- 1. Module A: Programming Basics - Python
- a. Installing Jupiter Notebooks
- b. Python Overview
- c. Python various Operators and Operators Precedence
- d. Getting input from user, comments, Multi line comments
- 2. Module B: Making Decisions and Loop - Python
- a. Types of Operators
- b. Data Types
- c. Flow Controls (Loops)
- d. Functions
- e. List compressors
- 3. Module C: List,Tuples,Dictionaries– Python
- a. Python Lists,Tuples,Dictionaries
- b. Accessing Values
- c. Basic Operations
- d. Indexing, Slicing, and Matrixes
- e. Built-in Functions & Methods
- 4. Module D: Functions And Modules – Python
- a. Introduction To Functions – Why
- b. Defining Functions
- c. Calling Functions
- d. Functions With Multiple Arguments.
- e. Anonymous Functions - Lambda
- 5. Module F: Introduction of Essential Python Libraries for Data Science
- a. Numpy
- b. Pandas
- c. Matplotlib
- d. Scikit-learn
- e. Seaborn
- 6. Module G: Numpy Package
- a. Importing Numpy
- b. Numpy overview
- c. Numpy Array creation and basic operations
- d. Indexing and Slicing
- e. Iterating over array
- f. Array manipulation
- g. Numpy universal functions
- h. Shape Manipulation
- i. Stacking and Splitting Arrays
- j. Indexing: Arrays of Indices, Boolean Arrays
- 7. Module H: Pandas Package
- a. Importing Pandas
- b. Pandas overview
- c. Object Creation: Series Object , Data Frame Object
- d. Handling the data and exporting the data
- e. Pandas Sorting
- f. Indexing, Selecting and filtering
- 8. Module I: Python Advanced: Data Mugging/Wrangling with Pandas
- a. Handling Missing Data (Fillna, Dropna, Replace, Interpolate etc.,)
- b. Group by Method
- c. Merging, Joining and Concatenating Data Frames
- d. Pivot Table
- e. Reshaping the Data Frame using melt
- f. Crosstab
- 9. Module J: Python Advanced: Visualization with Matplotlib and Seaborn
- a. Introduction to Matplotlib
- b. Creating basic chart : Line Chart, Bar Charts and Pie Charts
- c. Plotting from Pandas object
- d. Saving a plot
- e. Multiple Plots
- f. Plot Formatting : Custom Lines, Markers, Labels, Annotations, Colors
- g. Statistical Plots with Seaborn (Distribution Plots, Categorical Plots, Matrix and regression plots)
COURSE 3: UNDERSTANDING AND IMPLEMENTING MACHINE LEARNING
- 1. Module A: Introduction to Machine Learning
- a. What is Machine Learning
- b. Applications of Machine Learning
- c. Types of Machine Learning
- d. Machine Learning Process
- e. Python libraries suitable for Machine learning
- 2. Module B: Data Processing for Machine Learning
- a. What is data preprocessing
- b. Exploration of data (Uni-variate & Bi-variate analysis)
- c. Outlier Detection and Treatment
- d. Preprocess Data
- i. Formatting
- ii. Cleaning
- iii. Sampling
- e. Transform Data
- 3. Module C: Algorithms for Machine learning
- a. Supervised Learning Algorithms
- 1. Linear Regression
- i. Concepts and Application
- ii. Simple Linear Regression
- iii. Multivariate Linear Regression
- iv. Lasso Regression
- v. Ridge Regression
- 2. Logistic Regression – Concepts & Application
- 3. kNN – Concepts & Application
- 4. Decision Tree and random Forest – Concepts & Application
- 5. Support Vector Machines – Concepts & Application
- 6. Naïve Bayes – Concepts & Application
- 1. Linear Regression
- b. Unsupervised Learning
- i. k Means Clustering
- ii. Hierarchal Clustering
- a. Supervised Learning Algorithms
- 4. Module D: Dimensionality Reduction Techniques
- a. PCA – Principal Component Analysis
- b. LDA – Linear Discriminant Analysis
- 5. Module E: Other Topics
- a. K-fold Cross Validation
- b. Stratified Cross Validation
- c. Boosting Techniques
- i. Ada Boost
- ii. XG Boost